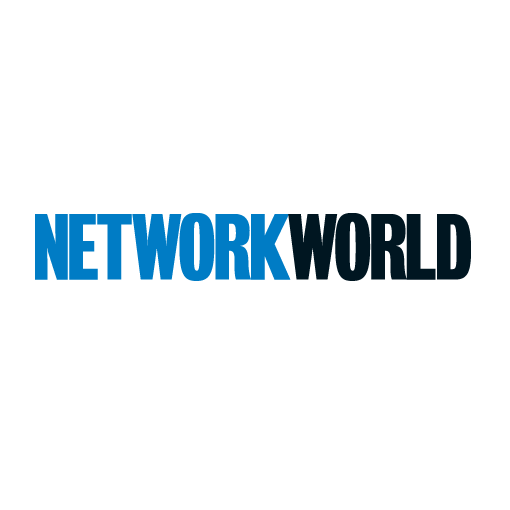
A Pentagon request for information that led to speculation about a nationwide 5G network created by a partnership between the mobile carriers and the government has provoked the wrath of Congressional leaders.
The controversy started with an official request for information from the Pentagon, which asks for guidance about the Department of Defense owning and operating 5G networks for domestic operations. Per Forrester vice president and research director Glenn O’Donnell, the plan as discussed would amount to a public-private partnership funded through government stimulus money and overseen by the DoD, but it would be implemented and operated by one of the country’s major wireless carriers.