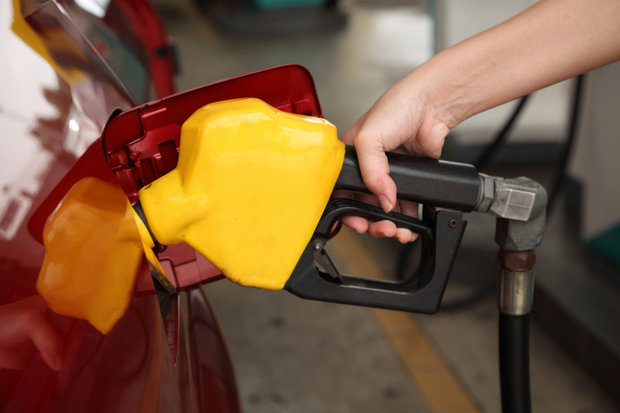
We’re hearing it everywhere. Machine learning and other types of artificial intelligence are the engines that will drive competitive advantage into the foreseeable future, but they have to rely on data for their fuel. Lots and lots data, but not just any data—they need high-quality, clean, up-to-date data that is accurately sourced and well managed.
One of the main challenges companies face is getting this data, and there are many reasons for that—ranging from the siloes that keep data trapped in organizations, to the fact that organizations might not be generating enough of this data or have a way to collect it in the first place. Some of the data they need might involve customer sentiment, manufacturing practices, or X-ray images.
On top of that, even if the organizations had the data, there are a lot of steps involved between data collection and getting the insights they need to answer a business question, such as “Why are we losing customers?” or “Why are some medical devices we are manufacturing defective?” Or, to predict an outcome such as “Are abnormalities in the X-ray images indicative of disease?”
After the data is collected, it needs to be cleansed, validated, and prepared to ensure that it is “good” data. This is the job of data engineers, who must also ensure that data management is designed, managed, and maintained properly. Then there are data scientists who create hypotheses that they will test through the AI systems—and, based on those, develop algorithms to teach the AI apps to look for patterns that can answer their questions. After this is accomplished, the work is continuously repeated, and never really done, because AI programs must continually learn and evolve.
This complex process brings us to another challenge. Data scientists are in short supply, and it’s only expected to get worse as the demand for AI increases along with data that is being collected from IoT devices and other sources. So, it is difficult for companies to build up all this expertise in-house even if they want to.
All of the challenges involved in collection, preparation, and maintenance of data and the ongoing development of AI algorithms has propelled a relatively recent business to the forefront: data-as-a-service, which involves outsourcing the various functions involved in these activities. We’ve all been hearing a lot about data-as-a-service, and with any new technology development, there’s lots of confusion and new terminology being tossed around. To simplify a technology service that is anything but, below I’ve broken down the data delivery services into two key offerings: model-as-a-service and insights-as-a-service—and there are different situations and times when each might be more applicable.
Model-as-a-service: the data and algorithms belong to you
In model-as-a-service, you would collect your own data, but turn to a provider to prepare it and develop the algorithms. This approach particularly makes sense if you have very customized or proprietary information. Imagine for example, if a company is trying to find out the likelihood of its customers leaving, a telecom provider is trying to determine the best way to cross-sell its products to customers, or a medical practitioner trying to determine if patients are likely to be readmitted after a hospital stay or take their prescribed medications. The answer to these questions—or predictions to these outcomes—can only accurately be based on your company-specific data.
In this approach, you not only own the data, but you own the algorithms as well. All the heavy lifting between sifting through the data, cleaning, preparing, monitoring, and maintaining it and all the ongoing work involved in developing algorithms or data models to answer your questions is done for you. And the insights you receive are yours. You gain competitive advantage based on your data, your customers, your company and your specific situation without the need of a data science department.
Buying the insights: insights-as-a-service
The other approach is insights-as-a-service in which you just gain the specific knowledge you are looking for, but nothing else. You don’t have to provide the data (although you could supplement some general data if you’d like), and you don’t own it or any of the algorithms that are developed. You just get the particular insight.
This makes sense if you’re looking for insight into a universal question that is not based on your particular set of data. For example, suppose a hedge fund is trying to determine if sales during the Christmas season are up. They could turn to an insights-as-a-service firm that might use satellite imagery and deep learning to count cars in shopping lots, for example. In this case, you will get the answer to your question, but that insight is available to other companies as well.
The good news is that despite the challenges in collecting and maintaining data, and developing algorithms—and the shortage of data scientists—you can still rely on AI to get the data insights you are looking for. Whether you need insight into your particular data through model-as-a-service or the answer to more universal questions outside your data set by turning to insights-as-a-service, the result is that data becomes the fuel that propels your business forward.
This article is published as part of the IDG Contributor Network. Want to Join?